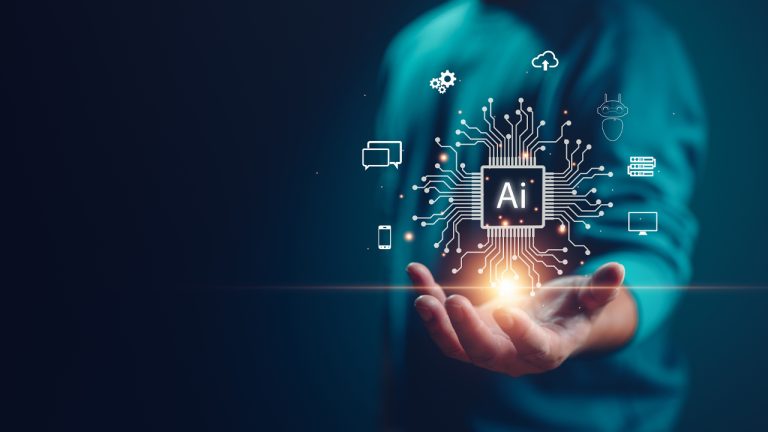
Explore the concept of prompt engineering, its significance, and how it works in fine-tuning language models.
Prompt engineering has become a powerful method for optimizing language models in natural language processing (NLP). It entails creating efficient prompts, often referred to as instructions or questions, to direct the behavior and output of AI models.
Due to prompt engineering’s capacity to enhance the functionality and management of language models, it has attracted a lot of attention. This article will delve into the concept of prompt engineering, its significance and how it works.
Prompt engineering involves creating precise and informative questions or instructions that allow users to acquire desired outputs from AI models. These prompts serve as precise inputs that direct language modeling behavior and text generation. Users can modify and control the output of AI models by carefully structuring prompts, which increases their usefulness and dependability.
Related: How to write effective ChatGPT prompts for better results
In response to the complexity and expanding capabilities of language models, prompt engineering has changed over time. Although quick engineering may not have a long history, its foundations can be seen in early NLP research and the creation of AI language models. Here’s a brief overview of the history of prompt engineering:
Prompt engineering was less common before the development of transformer-based models like OpenAI’s generative pre-trained transformer (GPT). Contextual knowledge and adaptability are lacking in earlier language models like recurrent neural networks (RNNs) and convolutional neural networks (CNNs), which restricts the potential for prompt engineering.
The introduction of transformers, specifically with the “Attention Is All You Need” paper by Vaswani et al. in 2017, revolutionized the field of NLP. Transformers made it possible to pre-train language models on a broad scale and teach them how to represent words and sentences in context. However, throughout this time, prompt engineering was still a relatively unexplored technique.
A major turning point for rapid engineering occurred with the introduction of OpenAI’s GPT models. GPT models demonstrated the effectiveness of pre-training and fine-tuning on particular downstream tasks. For a variety of purposes, researchers and practitioners have started using quick engineering techniques to direct the behavior and output of GPT models.
As the understanding of prompt engineering grew, researchers began experimenting with different approaches and strategies. This included designing context-rich prompts, using rule-based templates, incorporating system or user instructions, and exploring techniques like prefix tuning. The goal was to enhance control, mitigate biases and improve the overall performance of language models.
As prompt engineering gained popularity among NLP experts, academics and programmers started to exchange ideas, lessons learned and best practices. Online discussion boards, academic publications, and open-source libraries significantly contributed to developing prompt engineering methods.
Prompt engineering continues to be an active area of research and development. Researchers are exploring ways to make prompt engineering more effective, interpretable and user-friendly. Techniques like rule-based rewards, reward models and human-in-the-loop approaches are being investigated to refine prompt engineering strategies.
Prompt engineering is essential for improving the usability and interpretability of AI systems. It has a number of benefits, including:
Users can direct the language model to generate desired responses by giving clear instructions through prompts. This degree of oversight can aid in ensuring that AI models provide results that comply with predetermined standards or requirements.
Prompt engineering can be used as a tool to reduce bias in AI systems. Biases in generated text can be found and reduced by carefully designing the prompts, leading to more just and equal results.
Language models can be modified to display desired behaviors using prompt engineering. As a result, AI systems can become experts in particular tasks or domains, which enhances their accuracy and dependability in particular use cases.
Related: How to use ChatGPT like a pro
Prompt engineering uses a methodical process to create powerful prompts. Here are some crucial actions:
GPT-4 General Prompting Tips
— Chase Curtis (@realchasecurtis) April 2, 2023
The following tips will help give you a competitive advantage with the latest version of ChatGPT:
→ Capture Your Writing Style
Feed GPT a few samples of your writing and ask it to create a style guide for future outputs.
Example prompt:… pic.twitter.com/JWYYLV4ZLS
Establish the precise aim or objective you want the language model to achieve. Any NLP task, including text completion, translation and summarization, may be involved.
Clearly define the inputs required by the language model and the desired outputs you expect from the system.
Create prompts that clearly communicate the expected behavior to the model. These questions should be clear, brief and appropriate for the given purpose. Finding the best prompts may require trial and error and revision.
Put the created prompts to the test by feeding them into the language model and evaluating the results. Review the outcomes, look for flaws and tweak the instructions to boost performance.
Take into account the evaluation’s findings when calibrating and fine-tuning the prompts. To obtain the required model behavior, and ensure that it is in line with the intended job and requirements, this procedure entails making minor adjustments.
The firm behind ChatGPT is seemingly under pressure from other available open-source AI models and is prepping to enter the space with one of its own.
An open-source artificial intelligence (AI) model is reportedly being prepared for public release by OpenAI, the firm behind the AI chatbot ChatGPT.
In a May 16 report in The Information citing a person with knowledge of the plan, OpenAI is undertaking the move as pressure mounts from competing open-sourced AI models, such as those leaked from Meta in February.
The timeline of when the model would be released was not reported.
If OpenAI release a truly open-source model it would send progress stratospheric
— Alex MacCaw (@maccaw) May 15, 2023
It was said OpenAI’s open-source model would likely not be competitive with its flagship ChatGPT product, as the firm’s value comes from being able to sell access to its more sophisticated models.
OpenAI has faced stiff competition from open-source AI models such as Meta's LLaMa — which was originally limited to researchers but was leaked in full by a user from the imageboard site 4chan in late February.
Other open-source models include those from Stability AI, which opened its large language models in April, along with Databricks’ Dolly 2.0 AI, which it open-sourced days prior to Stability AI.
Open-source models mean the complete code is open to everyone. Anyone has the right to modify the models for any reason or fit them to specific purposes. Some firms choose to open-source their software as they believe it could benefit from contributions by outside developers.
Those building such models are getting significant backing funds too.
Related: MakerDAO publishes 5-phase roadmap featuring funding for open-source AI projects
On May 15, AI firm Together said it raised $20 million in a seed round backed by crypto figures including Oasis Labs co-founder Dawn Song, OpenSea co-founder Alex Atallah and Uniswap COO Mary-Catherine Lader — its stated mission is to provide open-source generative AI models.
Earlier in May, a leaked document from Google senior software engineer Luke Sernau pointed to open-source AI models as a significant threat to the company's own AI efforts.
“The uncomfortable truth is, we aren’t positioned to win this arms race and neither is OpenAI," Sernau wrote.
He added while Google was distracted by its competition with OpenAI, open-source AI models quietly became significantly more advanced. “They are lapping us," he wrote. “Open-source models are faster, more customizable, more private, and pound-for-pound more capable."
Cointelegraph contacted OpenAI for comment but did not immediately receive a response.
AI Eye: ‘Biggest ever’ leap in AI, cool new tools, AIs are the real DAOs
Update (May 16, 2:50 am UTC): This article has been updated to include more information from the leaked Google document and competing open-source AI models.